How does AI adapt to language changes and user intent ?
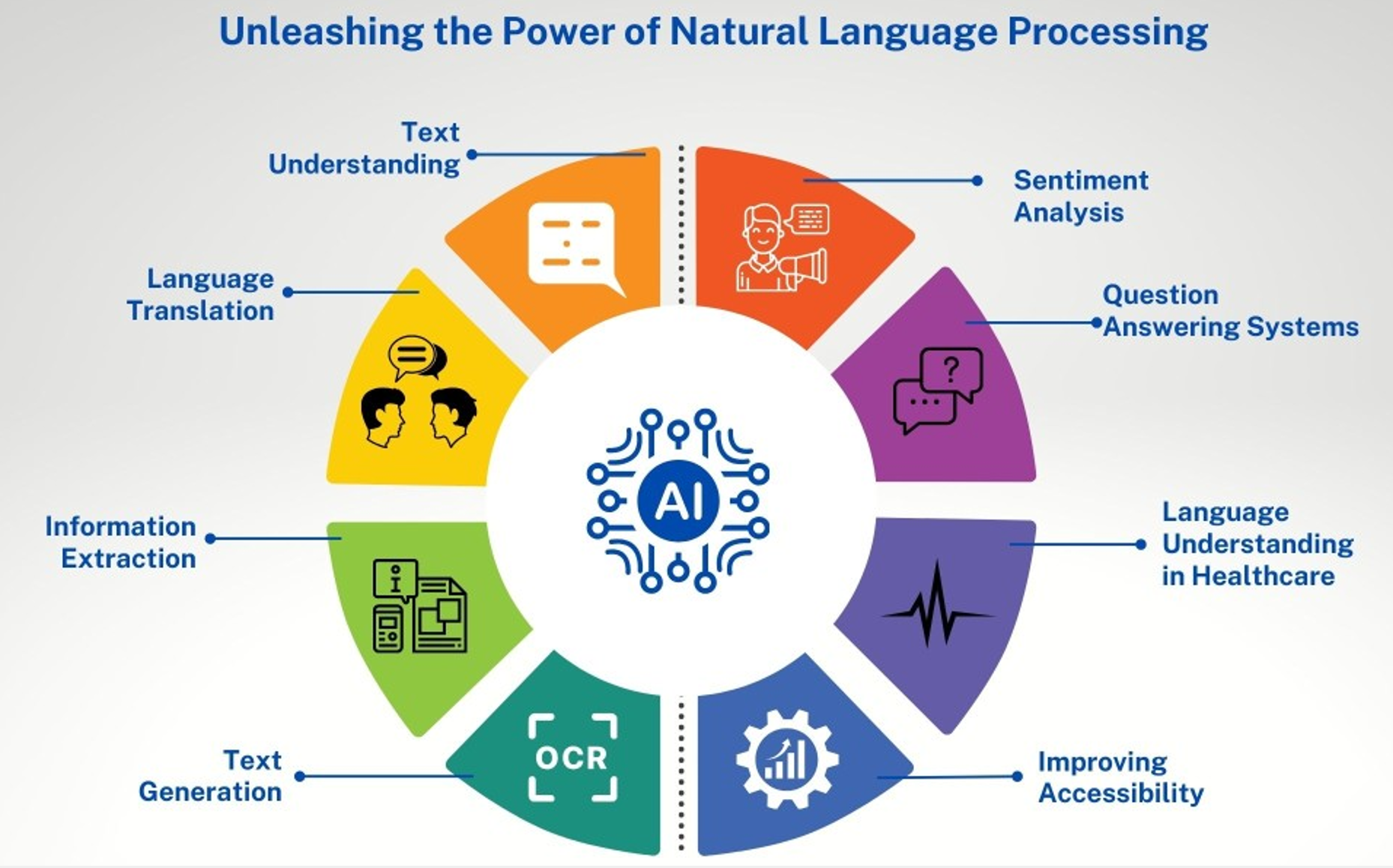
Artificial intelligence (AI) is transforming how we interact with technology. Its ability to adapt to evolving language patterns and accurately interpret user intent is a game-changer. Whether it’s recognizing industry-specific jargon or adjusting to user preferences, adaptive AI ensures more natural, human-like interactions.
But how does AI achieve this? It’s all about advanced natural language processing (NLP), real-time fine-tuning, and intent recognition pipelines. Let’s explore the core adaptation techniques, real-world use cases, and how AI answers questions and interprets information to enhance digital interactions.
Understanding AI adaptation to language and intent
AI adaptation to language involves refining its understanding to stay in sync with new words, changing expressions, and evolving communication styles. Intent recognition, on the other hand, focuses on understanding what the user truly wants, whether it’s asking for information or making a purchase.
By combining these two components, AI can offer context-rich, highly relevant responses. This isn’t just a luxury; it’s essential for businesses aiming to deliver seamless user experiences.
Key techniques for AI language adaptation
Adapting to language changes is a complex process. It requires a combination of cutting-edge learning techniques, continual exposure to new data, and smart model architecture. Each technique plays a specific role in refining AI’s language capabilities.
Transfer learning and few-shot learning
Transfer learning allows AI to build on existing knowledge, reducing training time and improving accuracy. For instance, a model trained on general text can quickly adapt to specialized content in legal, medical, or financial sectors.
Few-shot learning is another breakthrough. With just a handful of examples, it enables AI to adapt fast, a must for handling rare languages or niche industry terms.
Continued pre-training and embedding alignment
In continued pre-training, models are exposed to domain-specific data for an extended period. This helps fine-tune their language understanding for specific fields like financial analysis or technical support.
Meanwhile, embedding alignment ensures that newly added words fit into the model’s existing vocabulary, improving consistency and coherence in responses.
Try Wiseone for free with a 7-day pro trial
no credit card required
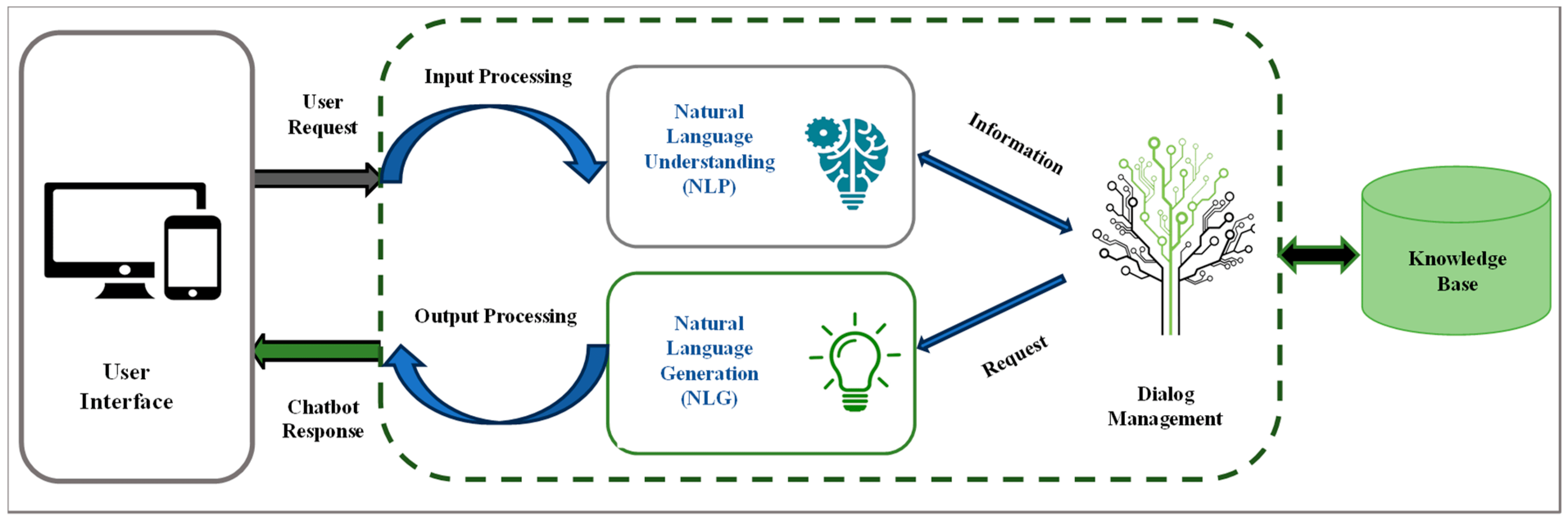
Intent recognition in AI systems
Intent recognition is about more than just understanding words. It’s about grasping the meaning behind them. AI systems use advanced NLP and machine learning models to predict user intent, even in ambiguous queries.
Successful intent recognition depends on how well the system can classify and respond to different intent categories—like requests, commands, or feedback. The better the intent detection, the more useful and precise the AI response.
Machine learning algorithms for intent classification
Modern intent recognition models rely on transformer-based architectures, which analyze both sentence structure and context. These models are trained using high-quality features, ensuring accurate predictions in complex scenarios.
Feature extraction and adaptive model training
Feature extraction focuses on identifying the most relevant data points in user input. It’s crucial for boosting the model’s efficiency. Adaptive training processes keep models updated, ensuring they evolve alongside user needs.
Enhancing AI adaptability
AI adaptability is about staying flexible. It involves dynamic adjustment processes that improve accuracy and keep models relevant over time. Techniques like self-calibration and multilingual adaptation help AI stay ahead of the curve.
Self-calibration and multilingual transformers
Self-calibrating models adjust their responses based on changing data patterns, improving both precision and recall. In parallel, multilingual transformers such as GPT-4 and BactrianX enable AI to adapt across languages, ensuring accurate responses in multilingual environments.
Learned Embedding Propagation (LEP)
Techniques like Learned Embedding Propagation (LEP) streamline adaptation processes. They reduce the need for retraining while maintaining high accuracy, making them cost-effective for large-scale deployments.
Applications of adaptive AI in language processing
Adaptive AI has a wide range of applications. From personalized customer service to real-time translation, these systems help businesses create seamless user experiences.
Advanced chatbots and virtual assistants
AI-powered chatbots handle multi-turn conversations, keeping track of context and improving customer support quality. In industries like banking and healthcare, these systems offer real-time assistance and reduce response times.
Hyper-personalized user experiences
Adaptive AI personalizes recommendations by analyzing behavioral data and sentiment. It provides tailored content suggestions on streaming platforms, e-commerce websites, and even educational tools.
Multilingual support for global businesses
Real-time language adaptation ensures smooth communication across borders. Adaptive AI helps companies localize content, improving interactions in legal, medical, and customer service settings.
Challenges and future directions
Despite its potential, adaptive AI faces several challenges. Developers must balance accuracy with efficiency while ensuring fairness and ethical practices. Emerging solutions are tackling these issues head-on.
Balancing accuracy and efficiency
Training models to adapt quickly while maintaining high accuracy can be resource-intensive. Techniques like model distillation and parameter-efficient tuning help reduce computational demands.
Addressing biases and ensuring fairness
Bias remains a significant concern in AI. Solutions like counterfactual fairness testing and diverse dataset augmentation help mitigate these risks and promote fairness.
Real-time adaptation and contextual learning
The future of AI adaptation lies in real-time learning. Soon, models will be able to update and adjust without full retraining, creating hyper-adaptive systems that respond instantly to changes in language and user behavior.
Final thought
AI’s ability to adapt to language evolution and shifting user intent is revolutionizing how we communicate with technology. By integrating transfer learning, multilingual transformers, and advanced intent recognition techniques, businesses can unlock the full potential of adaptive AI.
For tech lovers and professionals, adaptive AI isn’t just about efficiency. It’s about redefining innovation, making every interaction smarter and more personalized.
Join the Wiseone community
They’re talking about us on social media.